CENIIT PROJECT: Executable models for drug development
Elin Nyman
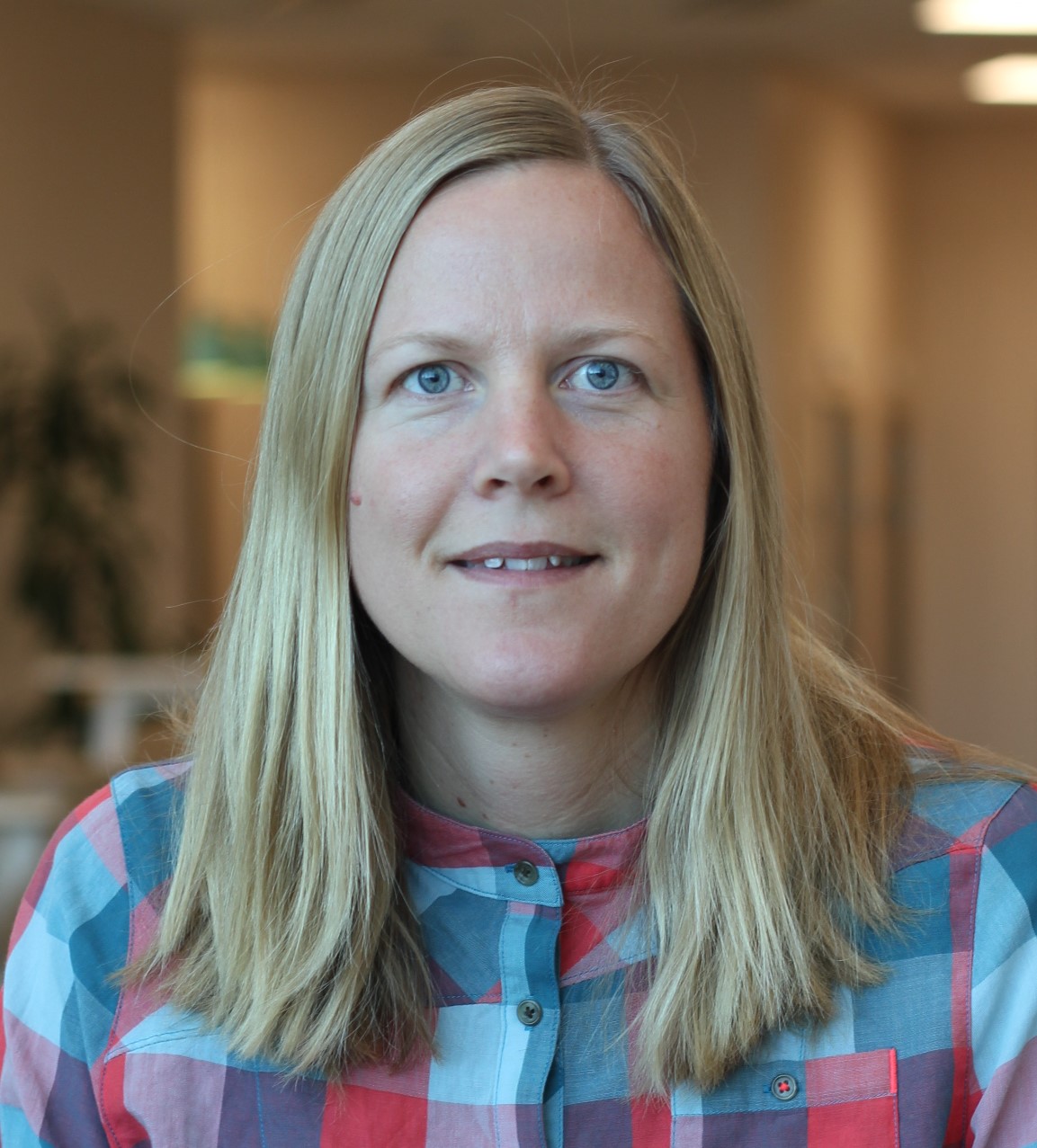
A major challenge for drug development is to handle and make optimal use of increasing amounts of large scale omics data. Omics data consists of measurement of e.g. all genes, all transcripts, or all proteins in a sample. The analysis of omics data is today mostly done within the field of bioinformatics, where data is mapped to static networks, often using known interactions. Methods within bioinformatics cannot be used to simulate time-resolved scenarios, e.g. to predict effects of new drugs. Such methods also lack the ability to integrate data from several sources into a consistent body of knowledge. This is instead the strength of executable models.
During the last 10 years, I have developed award-winning executable models (e.g. Nyman 2011, Nyman 2014, Rajan 2016) which have turned out to be highly useful for several major drug companies, such as AstraZeneca and Sanofi. AstraZeneca financed my first postdoc so that I could continue to work with model development, and also to work closer to their actual drug development projects. My postdoc period at AstraZeneca was awarded with an internally highly prestigious Science Prize – for the first time given to a theoretical postdoc. More recently, in a VR-financed postdoc at Harvard Medical School, I used data from cancer tumors during drug treatment, to improve executable-modeling methods to include more data for the drug response (Nyman et al 2019). Since executable models can be used to simulate scenarios other than the scenarios used for training, my developed methods could predict the response of new drug candidates and rank their ability to overcome drug resistance. The highest ranked drugs were validated, i.e. shown experimentally to effectively kill cancer cells and thereby reduce tumor growth. However, this approach does not scale to omics measurements, due to quadratic growth of the complexity of the problem with respect to the amount of measured variables. The goal of this project is therefore to combine the strength of executable models with omics data to be used in a new knowledge-driven framework for drug development.
Modeling Framework Development
To scale executable models to large scale omics measurements, we need a rethinking of current methods for parameter estimation: the problem must be decomposed into mini-problems that can be solved in parallel at a low computational cost (Figure 1A.). Such pairwise models will be based on knowledge from the largest pathway database for protein interactions in human tissue – Pathway Commons. We will extract biomedical interactions that maps to the information we have in data. This will lead to a long list of potential interactions, and we will use the pairwise models to sort out which interactions that are induced in the specific tissue sample. The pairwise models are based on ordinary differential equations. The parameters of the pairwise models will be estimated using an objective function to measure the agreement between simulations and data as the residual sum of squares (Figure 1B.). All pairwise models that are in agreement with data will be combined into a single network model (Figure 1C.). This model is predicted to be in fair agreement with data, since all pairwise models were fitted to data. The problem is instead that the model might be overfitted, since too many parameters have been allowed from the pairwise step, e.g. since overlapping pathways might have been introduced when the pairwise models are combined. Instead of analyzing the details of overlapping pathways, we will apply regularization to the full model, to reduce the number of interactions and avoid overfitting (Figure 1D.). For model validation, we will introduce alterations into the model and simulate the response (Figure 1E.). These responses will be compared with corresponding experimental data with gene edits from a so called CRISPR-Cas9 system developed at AstraZeneca. The model will either be in agreement with validation data, or rejected, and reiterated with the use of new knowledge obtained. In the latter case, new validation data will be gathered with other gene edits/combinations of gene edits. This iterative approach will be repeated until we have a model of high quality that cannot be rejected.
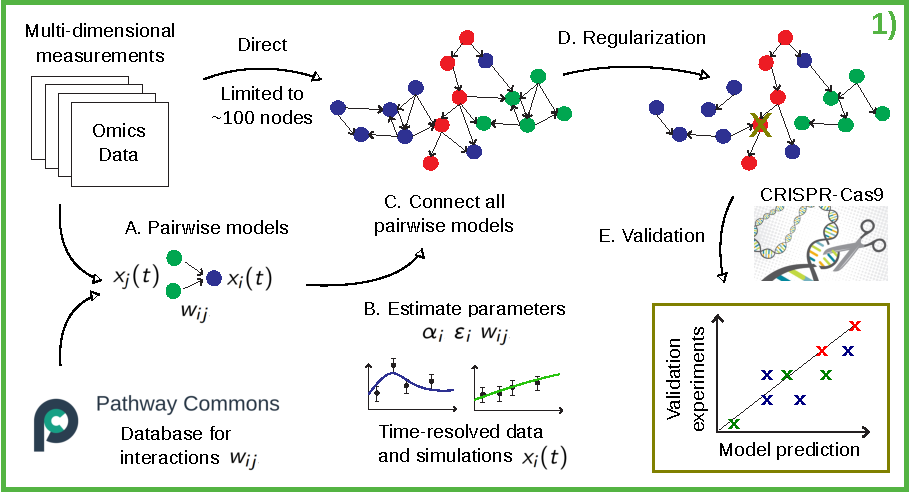
References
Nyman E, Stein RR, Jing X, Wang W, Marks B, Zervantonakis, IK, Korkut A, Gauthier NP, Sander C. Perturbation biology links temporal protein changes to drug responses in a melanoma cell line, bioRxiv 2019, doi: 10.1101/568758.
Rajan MR, Nyman E, Brännmark C, Olofsson CS, Strålfors P. Inhibition of FOXO1 transcription factor in primary human adipocytes mimics the insulin-resistant state of type 2 diabetes. Biochem J. 2018 May 31;475(10):1807-1820. doi: 10.1042/BCJ20180144.
Rajan MR, Nyman E, Kjölhede P, Cedersund G, Strålfors P. Systems-wide experimental and modeling analysis of insulin signaling through FOXO1 in human adipocytes, normally and in type 2 diabetes. J Biol Chem. 2016 Jul 22;291(30):15806-19. doi: 10.1074/jbc.M116.715763. Epub 2016 May 20.
Sips FL, Nyman E, Adiels M, Hilbers PA, Strålfors P, van Riel NA, Cedersund G. Model-based quantification of the systemic interplay between glucose and fatty acids in the postprandial state. PLoS One. 2015 Sep 10;10(9):e0135665. doi: 10.1371/journal.pone.0135665.
Nyman E, Rajan MR, Fagerholm S, Brännmark C, Cedersund G, Strålfors P. A single mechanism can explain network-wide insulin resistance in adipocytes from obese patients with type 2 diabetes. J Biol Chem. 2014 Nov 28;289(48):33215-30. doi: 10.1074/jbc.M114.608927.
Brännmark C, Nyman E, Fagerholm S, Bergenholm L, Ekstrand EM, Cedersund G, Strålfors P. Insulin signaling in type 2 diabetes: experimental and modeling analyses reveal mechanisms of insulin resistance in human adipocytes. J Biol Chem. 2013 Apr 5;288(14):9867-80. doi: 10.1074/jbc.M112.432062.
Nyman E, Brännmark C, Palmér R, Brugård J, Nyström FH, Strålfors P, Cedersund G. A hierarchical whole-body modeling approach elucidates the link between in vitro insulin signaling and in vivo glucose homeostasis. J Biol Chem. 2011 Jul 22;286(29):26028-41. doi: 10.1074/jbc.M110.188987.